A Data Driven Approach to Valuing Startups
The start-up economy has grown exponentially since the start of the millennium supported by unprecedented levels of investment and trends like the private IPO.
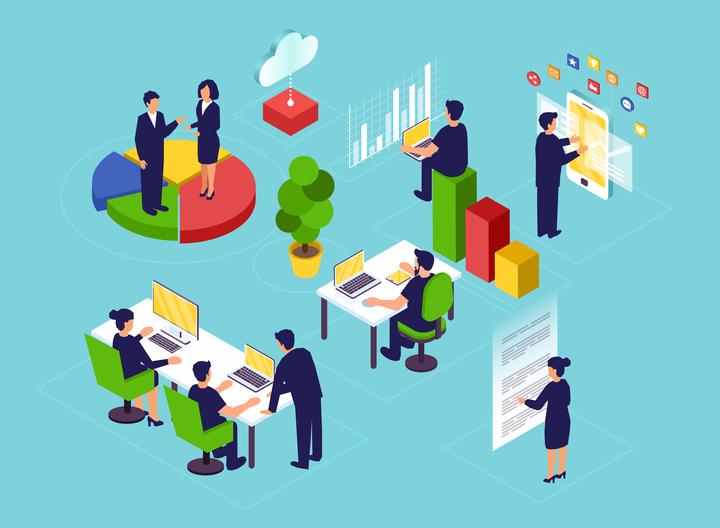
The start-up economy has grown exponentially since the start of the millennium supported by unprecedented levels of investment (think flagship funds like the SoftBank Vision Fund) and trends like the private IPO (companies staying private for longer). Private market failures and soaring valuations (e.g. Theranos, Juicero, WeWork) have put the spotlight on a previously smaller part of the financial markets. Private companies now impact everyone making it crucial to focus on multiple methods of valuation.
Private company valuation is difficult and subjective. In contrast to public companies, private companies do not have a readily available proxy for value such as market capitalisation. Current approaches involve both intrinsic methods (forecasting revenues and costs in discounted cash flows) and extrinsic methods (market-based comparison to similar listed companies or related M&A transactions). Data driven approaches have been limited to date due to the availability of data (funding rounds are not regularly disclosed) and the subjectivity in defining value.
My research combined intrinsic inputs (e.g. company revenue, founders, investors) with extrinsic market pricing outputs (e.g. funding rounds and M&A events) in order to develop a hybrid approach that calculates the aggregate market view of how a company with a given set of attributes is priced. The 3 approaches were set out as follows:
- Estimating a company’s next round of funding followed by an estimation of post-money valuation using S. Quintero’s regression model
- Estimating post-money valuation directly based on a recent fund round
- Using M&A deal values
The 3 approaches perform significantly better than naive models (predicting average values) with accuracies of 94.8%, 95.9% and 94.6% in comparison to 87.1%, 85.9%, 90.2% for approaches (1), (2), and (3). The most important feature was the numeric value representing the stage of funding for a given company (i.e series A-I); the well known correlation between stage of funding and amount of funding meant this result was expected. Interestingly Crunchbase Company Ranking was also important reflecting the strength of Crunchbase’s proprietary algorithms.
The next feature of importance was Total Equity Funding, which was much higher for companies at series A - I than seed, reflecting the lower funding totals for early stage companies. Other features of importance included Estimated Revenue Range and Active Tech Count. Technologies and patents have previously been shown to correlate with value (e.g. S. Hoenen showed that having pending patents is correlated with a higher first round of funding) and therefore supported previous research. Y Combinator was the most significant individual investor; this probably reflected the relative volume of transactions in the dataset, which made it easier for the model to draw relationships for this particular investor.
The results show that data driven approaches could be used as a valuable addition to support current industry accepted approaches for valuation. In particular for venture capital (VC) funds, operators and auditors. VCs could use the model to triage investment opportunities or to probe discussion through review of feature importances. Audit professionals could benefit from having an independent tool to support the mitigation of anchoring biases. Overall, there are lots of exciting opportunities for future work including incorporating other data sources (e.g. Pitchbook and Companies House) and further features (e.g. information on founders and investor ranking scores).
By Archie Judd
Linkedin Profile